Aerosols are critical vehicles for airborne pathogen transmission between the environment and humans. Timely and accurate detection of airborne pathogens is essential to contain infectious disease outbreaks at their source and to reduce nosocomial infection rates. Traditional pathogen detection methods often suffer from slow detection speeds, low sensitivity, and complicated procedures. Single-cell Raman spectroscopy, which can characterize the phenotypic features of microorganisms and offers advantages such as rapid, culture-free, and multi-target detection, has emerged as a promising technology for rapid pathogen identification. In recent years, the rapid development of artificial intelligence has further enhanced the resolution of Raman spectroscopy, enabling better differentiation of subtle spectral variations between bacteria. However, in real-world environments, where microbial diversity is vast and most species cannot be cultured, it remains a significant challenge to establish comprehensive Raman spectral databases for all environmental microorganisms and achieve fast and accurate identification of pathogens from complex airborne microbial communities.
To address this challenge, the team led by Prof. Zhu Yong-Guan and Cui Li from Institute of Urban Environment, Chinese Academy of Sciences (IUE, CAS) published a novel airborne pathogen detection technology combining single-cell Raman spectroscopy with an open-set deep learning algorithm on Jan 8th inScience Advances. The researchers built a Raman spectral database for pathogens in aerosols and introduced an open-set loss function, optimization thresholds within the deep learning algorithm. This approach enabled rapid and accurate identification of five key airborne pathogens in complex, real-world environments. For air samples with pathogen abundances exceeding 1%, the entire process, including sample collection, preprocessing, identification and reporting, takes only one hour. The system achieves an impressive 93% average identification accuracy for five target pathogens, with a 36% reduction in false positive rates compared to traditional close-set algorithms. Detection sensitivity reaches the single-cell level. The method is also capable of targeting pathogens even in real-world air samples containing over 4,600 microbial species, demonstrating a significant improvement in resistance to interference compared to traditional methods. The method has been further validated in various real-world settings, including hospitals, shopping malls, dinning halls, kitchen waste plants, microbiology labs, and public lavatories. This method overcomes the issues of slow detection and poor identification performance of traditional techniques, providing a powerful tool for environmental biosafety monitoringand early warning systems, as well as for preventing the airborne transmission of pathogens.
Assoc. Prof.Zhu Longji from IUE, is the first author of the paper, while Prof. Zhu Yong-Guan and Cui Li are co-corresponding authors. Prof. Tian Zhong-Qun, Ren Bin (Xiamen University), Assoc. Prof. An Zhulin (Institute of Computing Technology, CAS), Prof. Peter J. Vikesland (Virginia Tech University), and Prof. Francis L. Martin (UK) provided guidance of the work. The research was supported by the National Natural Science Foundation of China (42021005, 32100083, 22176186), the National Key R&D Program of China (2022YFF0713100), and the Chinese Academy of Sciences (ZDBS-LY-DQC027).
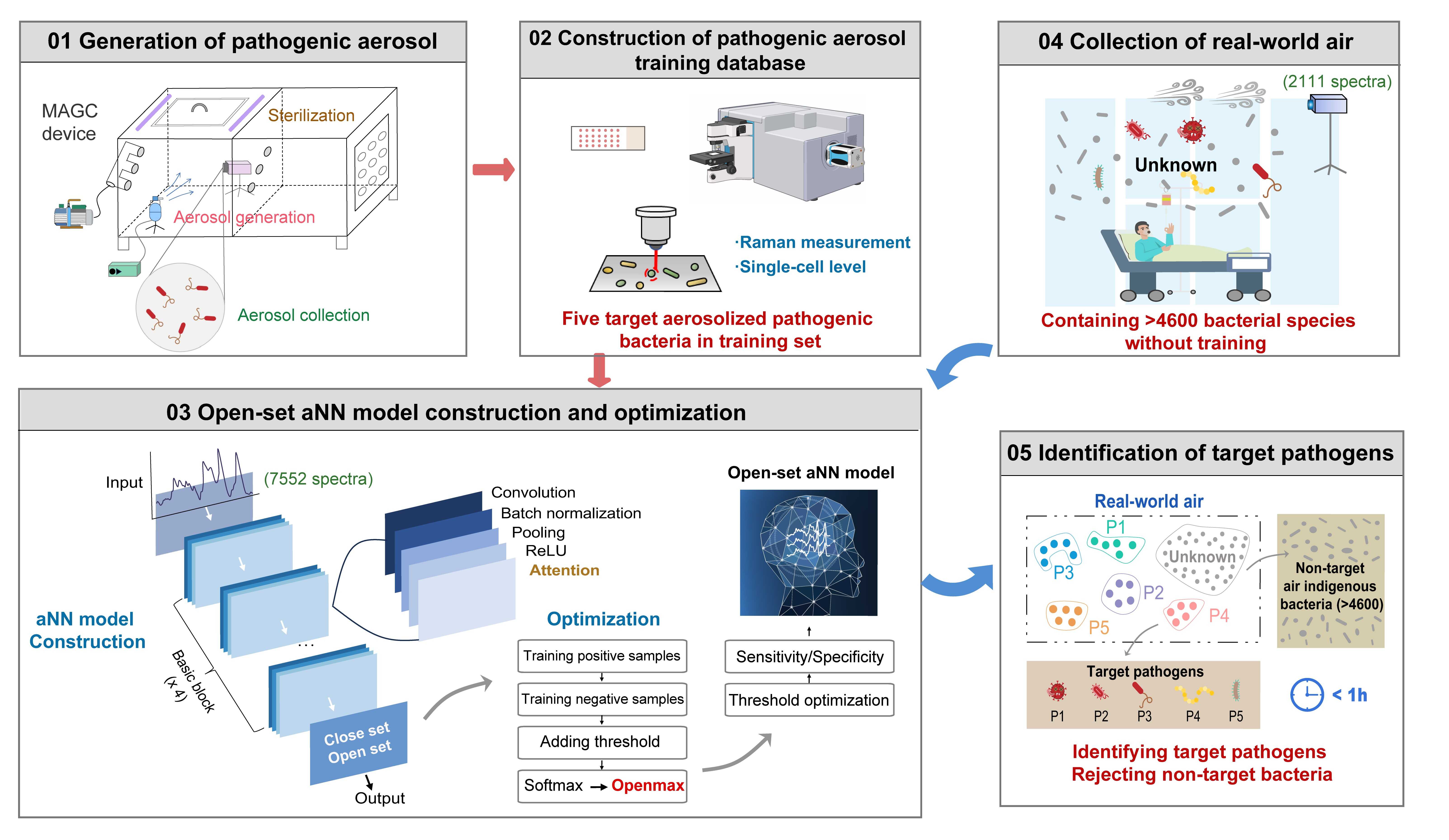
Figure 1: Workflow of the Methodology (Figure by Zhu et al.)
Contact:
Zhu Longji
Institute of Urban Environment, Chinese Academy of Sciences
E-mail: ljzhu@iue.ac.cn