Unlike previous studies focused on the energy use and carbon emissions of AI models, this study has developed a novel approach, termed as computational power-driven material flow analysis (CP-MFA). This approach aims to quantify and explore strategies for managing the electronic waste (e-waste) generated by GAI, with a specific emphasis on large language models.
Projections from the study suggest that the proliferation of generative AI could lead to an additional 5 million tonnes of e-waste by 2030. These estimates vary based on different scenarios of GAI expansion, computing resource requirements, and their operational lifespans. The research indicates a potential thousandfold increase in annual generative AI e-waste, surging from 2,600 tonnes in 2023 to 400,000-2,500,000 tonnes annually by 2030. This is equivalent to each individual on the planet discarding between 0.2 to 1.6 iPhones by 2030.
To tackle such challenge, this study proposed a set of circular economy strategies, like improving performance, increasing lifespan, and re-using modules in manufacturing, and further explored the potential of those strategies in reducing this e-waste. It is estimated that around 16–86% of those e-waste can be avoided with combined efforts from the GAI value chain.
The researchers also underscore the impact of geopolitical tensions within the semiconductor industry, which could exacerbate the e-waste predicament. In a scenario where nations face challenges in acquiring the latest chips for their AI technologies, they may resort to older models that are swiftly discarded.
“The objective is not to impede the adoption of this transformative GAI technology”, emphasized by Dr. Peng Wang and the research team, “they recognize as both promising and increasingly inevitable in our modern world”. Rather than hinder its progress, said by Peng, “the primary goal is to prepare the global community for the tangible impacts of its rapid expansion”.
This study is supported by the National Natural Science Foundation of China and the CAS IUE Research Program, with Dr. Peng Wang and Ling-Yu Zhang leading this work, complemented by contributions from Dr. Asaf Tzachor and Dr. Wei-Qiang Chen as corresponding authors.
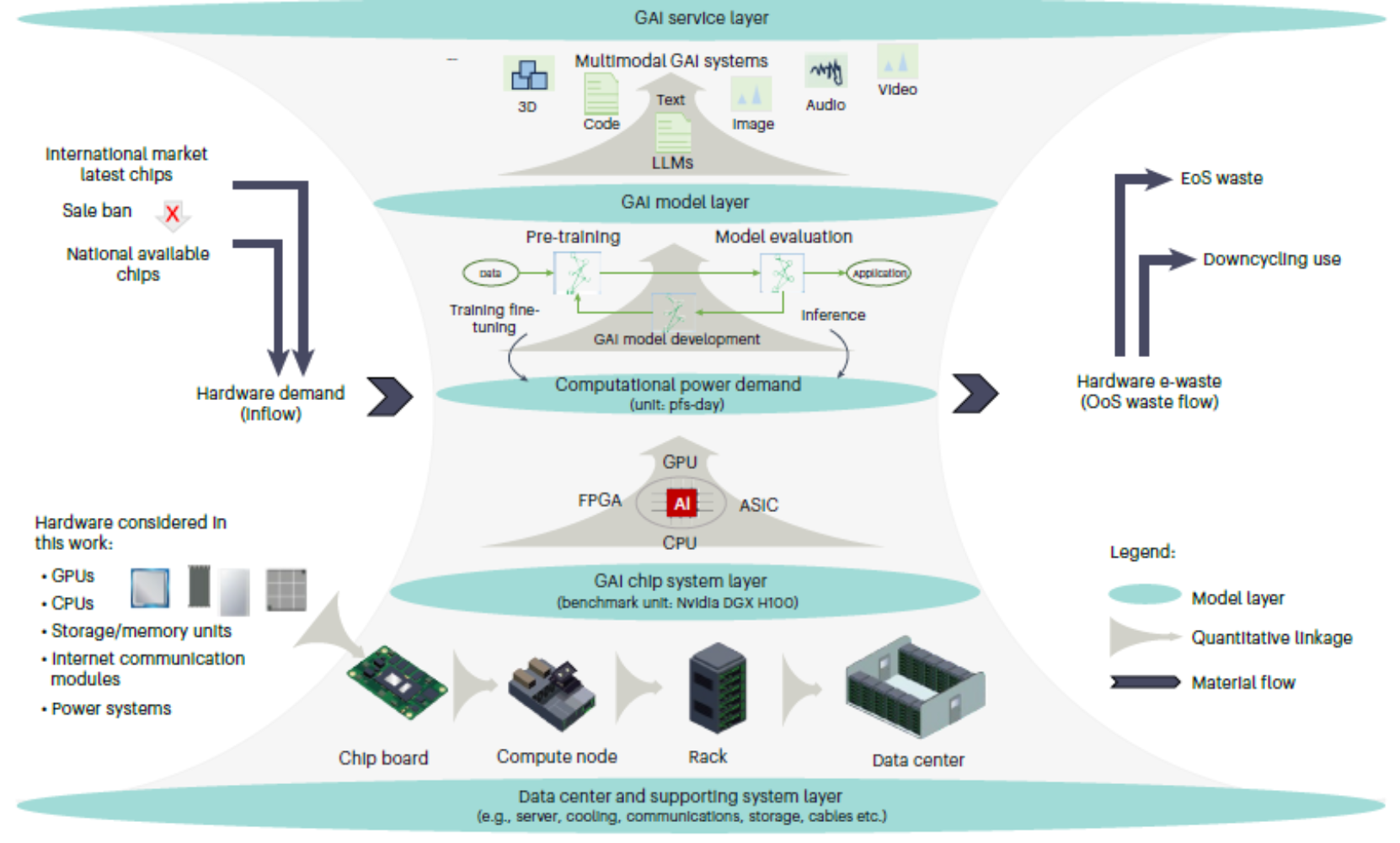
Figure 1 Hierarchical framework of the computational power-driven material flow analysis model